Until recently, SQL IN
and EXISTS
were almost exactly the same to me. There is a significant difference both in execution plans and time of execution though, as I found out after not being able to speed up my workmate’s query.
Assume two not-as-small-as-they-might-be tables:
BEGIN;
CREATE UNLOGGED TABLE test.small AS
SELECT * FROM generate_series(0, 500000) id;
CREATE UNLOGGED TABLE test.big AS
SELECT (random() * 4000000)::integer id
FROM generate_series(0, 4000000);
COMMIT;
To find out what rows from test.big
is missing in test.small
, you’ll use one of these queries:
SELECT id
FROM test.big
WHERE id NOT IN (SELECT id FROM test.small);
QUERY PLAN
-----------------------------------------------------------------------------------------
Seq Scan on big (cost=8463.01..42313.02 rows=1000000 width=4) (actual time=177.061..864.043 rows=1500894 loops=1)
Filter: (NOT (hashed SubPlan 1))
Rows Removed by Filter: 499107
SubPlan 1
-> Seq Scan on small (cost=0.00..7213.01 rows=500001 width=4) (actual time=0.045..34.727 rows=500001 loops=1)
Total runtime: 904.413 ms
(6 rows)
SELECT id
FROM test.big
WHERE NOT EXISTS (
SELECT 1
FROM test.small
WHERE test.big.id = test.small.id
);
QUERY PLAN
-----------------------------------------------------------------------------------------
Hash Anti Join (cost=15417.02..82100.58 rows=955189 width=4) (actual time=100.257..1240.343 rows=1500894 loops=1)
Hash Cond: (big.id = small.id)
-> Seq Scan on big (cost=0.00..28850.01 rows=2000001 width=4) (actual time=0.016..125.024 rows=2000001 loops=1)
-> Hash (cost=7213.01..7213.01 rows=500001 width=4) (actual time=100.068..100.068 rows=500001 loops=1)
Buckets: 65536 Batches: 2 Memory Usage: 8800kB
-> Seq Scan on small (cost=0.00..7213.01 rows=500001 width=4) (actual time=0.011..35.543 rows=500001 loops=1)
Total runtime: 1280.609 ms
That’s not a significant difference in time execution, is it?
What if you want to find out what rows from test.small
is missing in test.big
?
SELECT id
FROM test.small
WHERE id NOT IN (SELECT id FROM test.big);
QUERY PLAN
---------------------------------------------------------------------------
Seq Scan on small (cost=0.00..12915788669.52 rows=250000 width=4)
Filter: (NOT (SubPlan 1))
SubPlan 1
-> Materialize (cost=0.00..46663.01 rows=2000001 width=4)
-> Seq Scan on big (cost=0.00..28850.01 rows=2000001 width=4)
(5 rows)
SELECT id
FROM test.small
WHERE NOT EXISTS (
SELECT 1
FROM test.big
WHERE test.big.id = test.small.id
);
QUERY PLAN
-------------------------------------------------------------------------
Hash Anti Join (cost=61663.02..180597.23 rows=1 width=4)
Hash Cond: (small.id = big.id)
-> Seq Scan on small (cost=0.00..7213.01 rows=500001 width=4)
-> Hash (cost=28850.01..28850.01 rows=2000001 width=4)
-> Seq Scan on big (cost=0.00..28850.01 rows=2000001 width=4)
(5 rows)
It took me ~750 ms to get the result with EXISTS
expression. I kept IN
running whole night with no result. I’m not really sure why IN
is so much slower, it might be caused by checks for NULL
values. The speed is also related to the size of the subquery, thus the difference when tables were switched.
LEFT JOIN
can be used to achieve the same result, I find its syntax less obvious though.
No indexes were built this time, I know they don’t help the IN
performance at all from my previous tests. Tested with PostgreSQL 9.3.9.
As mentioned in QGIS Tips For Collaborative Mapping we’re in the middle of crop evaluation project at CleverMaps.
With the QGIS workflow up and running, I’ve been focused on different QGIS related task: automatic map generation for land blocks that meet certain conditions. The logic behind identifying such land blocks is as follows:
- if the original area and the measured one differ more than 0.5 % or
- number of declared crops differs from number of crops identified or
- at least one parcel in the land block was given a certain error code
Let’s assume that with several lines of SQL code we can store these mentioned above in a table called land_blocks
with geometries being the result of calling ST_Union()
over parcels for each land block.
Map composition
Every map should feature following layers:
- land blocks (remember the
land_blocks
table?) - labeled with ID, yellowish borders, no fill
- land parcels - that’s my source layer - labeled with letters, blue borders, no fill
- other layers
- HR, VHR, NIR imagery, orthophoto - served via WMS
Labels should be visible only for the featured land block (both for the land parcels and the land block itself. The whole map scales dynamically, showing small land blocks zoomed in and the large ones zoomed out.
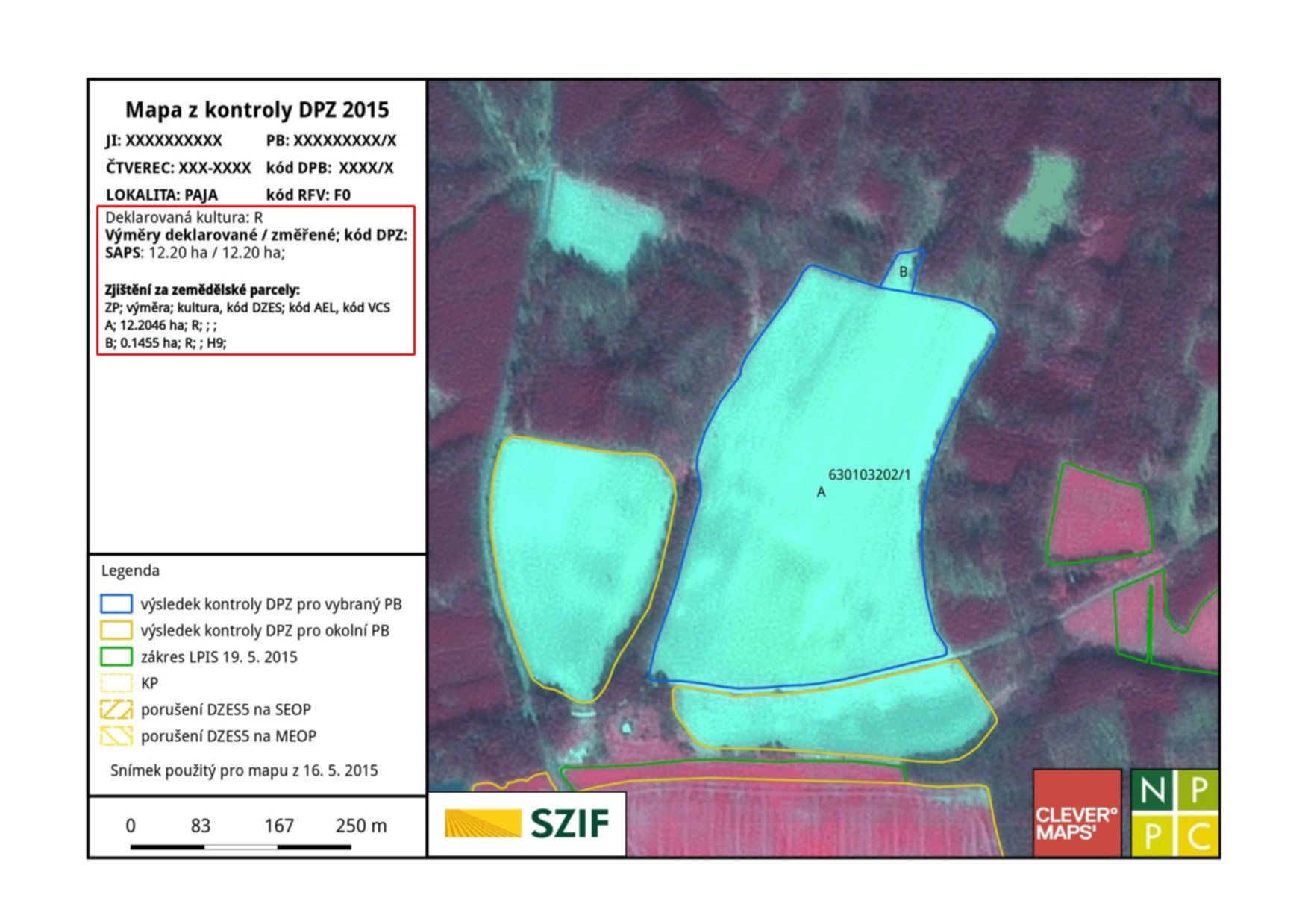
Every map features additional items:
- dynamic list of subsidies farmer asks for - showing both measured and declared area
- dynamic list of land parcels with their areas and error codes
- scalebar
- map key
- logos
Atlas creation
Now with requirements defined, let’s create some maps. It’s incredibly easy to generate a series of maps with QGIS atlas options.
Atlas generation settings
Coverage layer is presumably the only thing you really need - as the name suggests, it covers your area of interest. One map will be created for each feature in this layer, unless you decide to use some filtering - which I did.
Output filenames can be tweaked to your needs, here’s what such a function might look like. If there is a slash in the land block ID (XXXXXXX/Y), the filename is set to USER-ID_XXXXXXX-00Y_M_00
, USER-ID_XXXXXXX-000_M_00
otherwise.
CASE WHEN strpos(attribute($atlasfeature, 'kod_pb'), '/') > -1
THEN
ji || '_' ||
substr(
attribute($atlasfeature, 'kod_pb'), 0,
strpos(attribute($atlasfeature, 'kod_pb'), '/')+1 -- slash position
) || '-' ||
lpad(
substr(
attribute($atlasfeature, 'kod_pb'),
strpos(attribute($atlasfeature, 'kod_pb'), '/') + 2,
length(attribute($atlasfeature, 'kod_pb'))
),
3, '0') || '_M_00'
ELSE
ji || '_' || attribute($atlasfeature, 'kod_pb') || '-000_M_00'
END
Map scale & variable substitutions
Different land blocks are of different sizes, thus needing different scales to fit in the map. Again, QGIS handles this might-become-a-nightmare-pretty-easily issue with a single click. You can define the scale as:
- margin around feature: percentage of the space displayed around
- predefined scale (best fit): my choice, sometimes it doesn’t display the entire land block though
- fixed scale: sets the scale the same for all the maps
With these settings, I get a map similar to the one below. Notice two interesting things:
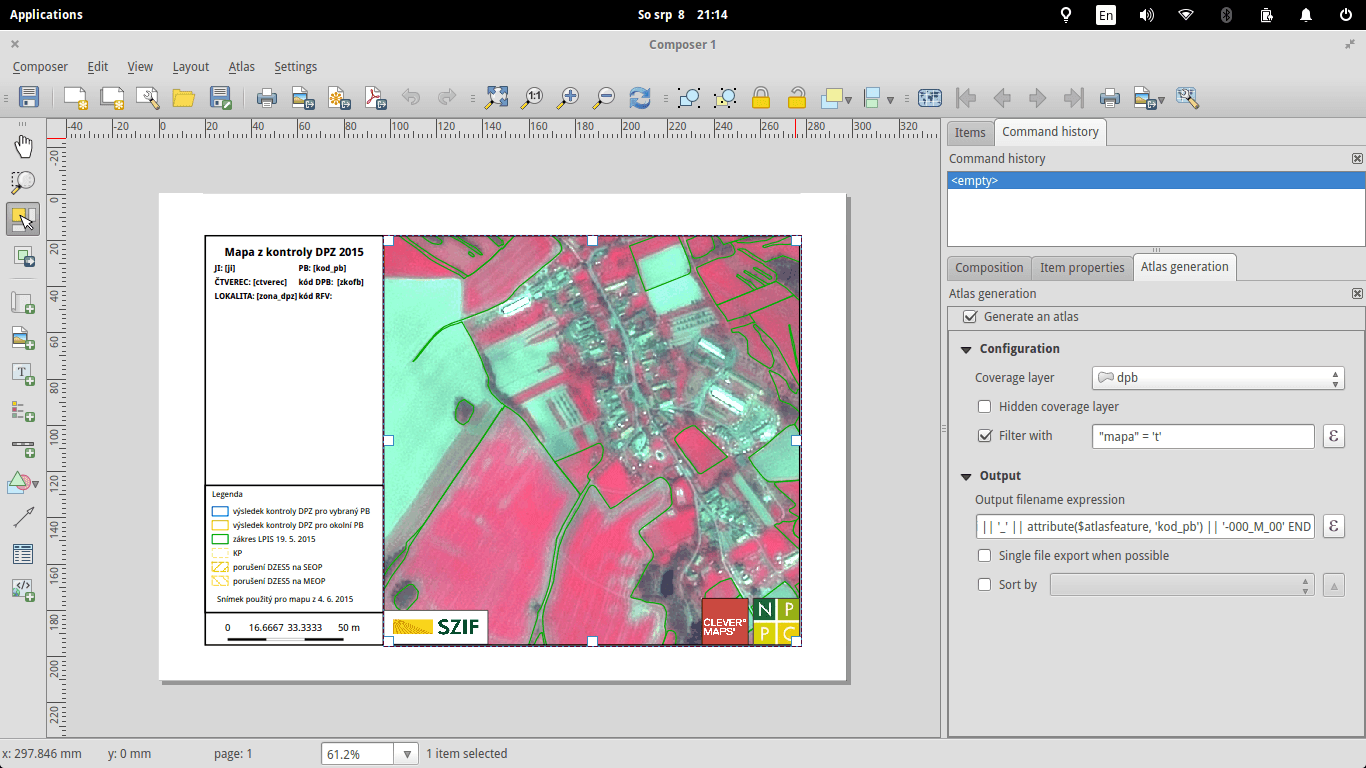
- Scale uses decimal places, which I find a huge failure. Has anyone ever seen a map with such scale? The worst is there is no easy way to hide these, or at least I didn’t find one.
- You can see a bunch of
[something in the brackets]
notations. These will be substituted with actual values during the atlas generation. Showing land block ID with a preceeding label is as easy as [%concat('PB: ', "kod_pb")%]
(mind the percentage sign).
Styling the map using atlas features
Atlas features are a great help for map customization. As mentioned earlier, in my case, only one land block label per map should be visible. That can be achieved with a simple label dialog expression:
CASE
WHEN $id = $atlasfeatureid
THEN "kod_pb"
END
QGIS keeps reference to each coverage layer feature ID during atlas generation, so you can use it for comparison. The best part is you can use IDs with any layer you need:
CASE
WHEN attribute($atlasfeature, 'kod_pb') = "kod_pb"
THEN "kod_zp"
END
With this simple expression, I get labels only for those land parcels that are part of the mapped land block. Even the layer style can be controlled with atlas feature. Land parcels inside the land block have blue borders, the rest is yellowish, remember? It’s a piece of cake with rule-based styling.
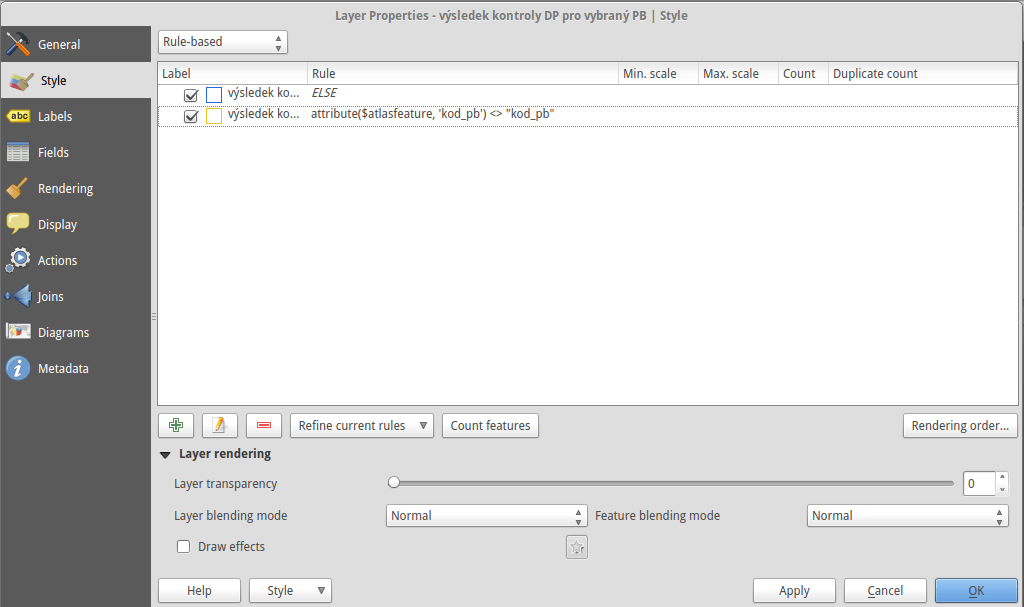
Atlas generation
When you’re set, atlas can be created with a single button. I used SVG as an output format to easily manipulate the map content afterwards. The resulting maps look like the one in the first picture without the text in the red rectangle. A Python script appends this to each map afterwards.
Roughly speaking, generating 300 maps takes an hour or so, I guess that depends on the map complexity and hardware though.
Adding textual content
SVG output is just plain old XML that you can edit by hand or by script. A simple Python script, part of map post-processing, loads SVG from the database and adds it to the left pane of each map.
SELECT
ji,
kod_pb,
concat(
'<g fill="none" stroke="#000000" stroke-opacity="1" stroke-width="1"
stroke-linecap="square" stroke-linejoin="bevel" transform="matrix(1.18081,0,0,1.18081,270.0,550.0)"
font-family="Droid Sans" font-size="35" font-style="normal">',
kultura, vymery, vymery_hodnoty,
vcs_titul, vcs_brk, vcs_brs, vcs_chmel,
vcs_zvv, vcs_zv, vcs_ovv, vcs_ov, vcs_cur, vcs_bip,
lfa, lfa_h1, lfa_h2, lfa_h3,
lfa_h4, lfa_h5, lfa_oa, lfa_ob, lfa_s,
natura, aeo_eafrd_text, dv_aeo_eafrd_a1,
dv_aeo_eafrd_a2o, dv_aeo_eafrd_a2v, dv_aeo_eafrd_b1,
dv_aeo_eafrd_b2, dv_aeo_eafrd_b3, dv_aeo_eafrd_b4,
dv_aeo_eafrd_b5, dv_aeo_eafrd_b6, dv_aeo_eafrd_b7,
dv_aeo_eafrd_b8, dv_aeo_eafrd_b9, dv_aeo_eafrd_c1,
dv_aeo_eafrd_c3, aeko_text, dv_aeko_a, dv_aeko_b, dv_aeko_c,
dv_aeko_d1, dv_aeko_d2, dv_aeko_d3, dv_aeko_d4, dv_aeko_d5,
dv_aeko_d6, dv_aeko_d7, dv_aeko_d8, dv_aeko_d9, dv_aeko_d10,
dv_aeko_e, dv_aeko_f, ez, dzes_text, rep, obi, seop, meop, pbz, dzes7,
'</g>'
) popis
FROM (...);
Each column from the previous query is a result of SELECT
similar to the one below.
SELECT concat('<text fill="#000000" fill-opacity="1" stroke="none">BrK: ', dv_brk, ' ha / ', "MV_BRK", ' ha;', kod_dpz, '</text>') vcs_brk
The transform="matrix(1.18081,0,0,1.18081,270.0,550.0)
part puts the text on the right spot. Great finding about SVG is that it places each <text>
element on the new line, so you don’t need to deal with calculating the position in your script.
Scale adjustment is done with a dirty lambda function.
content = re.sub(r">(\d{1,3}\.\d{3,5})</text>", lambda m :"> " + str(int(round(float(m.group(1))))) + "</text>", old_map.read())
SVG to JPEG conversion
We deliver maps as JPEG files with 150 DPI on A4 paper format. ImageMagick converts the formats with a simple shell command:
convert -density 150 -resize 1753x1240 input.svg output.jpg
Conclusion
I created pretty efficient semi-automated workflow using several open source technologies that saves me a lot of work. Although QGIS has some minor pet peeves (scale with decimal places, not showing the entire feature, not substituting variables at times), it definitely makes boring map creation quite amusing. The more I work with big data / on big tasks, the more I find Linux a must-have.
The whole process was done with QGIS 2.10, PostGIS 2.1, PostgreSQL 9.3, Python 2.7, ImageMagick 6.7.